Understanding How Machines Learn
For decades, there have been great hopes for advances in artificial intelligence. We have seen occasional waves of euphoria, but as often as not, each new florescence has been followed by another AI winter. The expectations of these new technologies were too high for science to ever have a real chance at meeting them.
A couple of years ago, all that changed. The breakthrough came about with developments in deep neural networks, one of the deep-learning techniques that are standard AI technology today. The rapid growth in processing power also contributed significantly to the AI revolution, making it possible to access enormous datasets that can be used to train these self-learning systems. But it isn’t yet entirely clear why deep learning is so effective. Gitta Kutyniok even goes so far as to call it a mystery—not exactly a common description for a mathematician to use.
What Do These Machines Need in the Way of Training Content?
Gitta Kutyniok holds the Bavarian AI Chair for Mathematical Foundations of Artificial Intelligence at LMU, one of the AI professorships funded under the State of Bavaria’s High-Tech Agenda. Her research work addresses precisely these types of questions: when systems automatically learn to make decisions based on numerous training examples, when they gain experience, systematize it, and use it to derive rules, we want to know how they arrive at these decisions. Kutyniok wants to find out what the main criteria are in these processes. Conversely, she also aims to identify the most important components that artificial neural networks and the resulting algorithms need in order to reach the “right” decisions, and what these machines need in the way of learning material. She also aims to unravel what guidelines need to be drafted and what the “ideal setup” for neural networks is, as well as how to ultimately maintain a practical understanding of what self-learning machines do—that is, how to maintain “explainable AI.”
“I Want to Make Neural Networks More Secure and Robust.”
This last issue is becoming increasingly important. After all, there are a number of potential applications of AI in highly sensitive areas, such as computer-aided diagnosis and treatment planning. “We don’t yet have a sufficient understanding,” Kutyniok says, “of how well neural networks actually work. For example, we have no error estimate for them.” It often happens in experiments that slight variations in the setup suddenly result in the system making glaring mistakes. That’s why Kutyniok formulates her research aim thus: “I want to make neural networks more secure, more robust.”
Combining the Best of Two Worlds
Kutyniok is also working on AI applications, primarily in medical imaging, a field in which she gained years of experience before turning to neural-network research. She discovered, for example, that by using conventional model-based techniques, such as compressed sensing, in combination with shearlets, she could develop methodologies that accelerate data acquisition in magnetic resonance tomography (MRT), “so patients don’t have to stay in the tube so long.” Shearlets are special function systems that make it possible to represent images particularly efficiently. Learning methods such as neural networks are good at detecting certain patterns in the original data. “In contrast, the inner workings of humans are almost too complex to permit precise mathematical modeling.” Kutyniok therefore pursues the strategy of combining the two approaches, physical models and AI techniques, thus, in her words, “uniting the best of both worlds.”
I have an outstanding research environment here. Many leading scientists are based in Munich, offering many opportunities for cooperation.Prof. Gitta Kutyniok, Bavarian AI Chair for Mathematical Foundations of Artificial Intelligence, LMU
Dual Degree
Kutyniok studied mathematics and computer science and obtained a degree in both disciplines. Following her Habilitation, she attended leading US universities Princeton, Stanford, and Yale on a Heisenberg Scholarship from the Deutsche Forschungsgemeinschaft, or DFG (German Research Foundation). Finally, she was awarded an Einstein Professorship for mathematics at Technische Universität Berlin. She has been in Munich since October 2020.
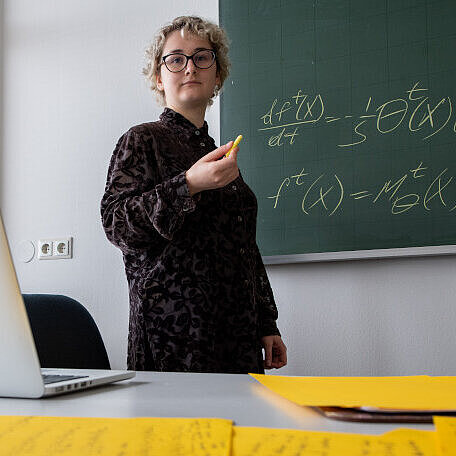
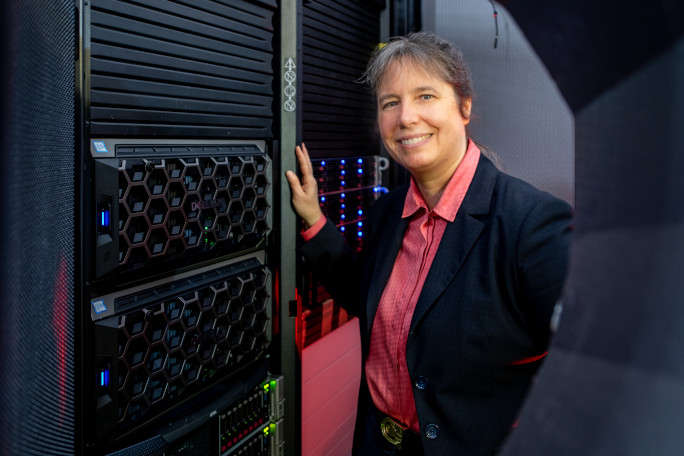
Mathematics, Informatics, and Statistics United within One Faculty
“I have an outstanding research environment here: Many leading scientists are based in Munich, offering many opportunities for cooperation at LMU, and even more if we also count the Technical University,” says Kutyniok. And as for the shape of her own research field, it lines up fairly precisely with the structures she encounters at LMU. “The faculty unites mathematics, computer science, and statistics. At most other universities, these disciplines are more widely scattered.”
The Right Base
Kutyniok recently obtained funding through a DFG-financed Priority Programme on the theoretical foundations of deep learning that is likewise expected to bring mathematicians, computer scientists, and statisticians together. As is common with this funding format, in the second phase, researchers from all over Germany can apply to participate with their project ideas. The selection processes are currently under way. “The aim of this approach is not only to pool research into deep learning, but also to contribute to something like community building in this field.”
Munich is probably the right base for this, too, reasons Kutyniok. After all, the State of Bavaria is boosting its High-Tech Agenda’s AI initiatives, which provided the financial and structural underpinnings for the German research landscape to join the race: “There is a dynamic emerging here that is unrivaled in Germany—or indeed anywhere in the world.”